Featured Case Studies
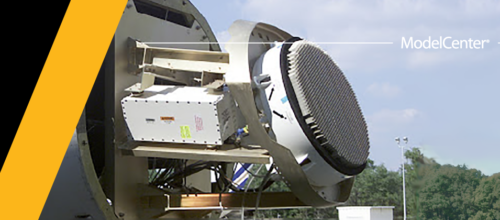
Northrop Grumman | Phased Array Antenna
Case Study
Northrop Grumman Corp. applied Model-Based Systems Engineering (MBSE) and Multidisciplinary Analysis and Optimization (MDAO) to the development of Phased Array Antenna Models to Improve Design Time and Quality
Northrop Grumman Corp. applied Model-Based Systems Engineering (MBSE) and Multidisciplinary Analysis and Optimization (MDAO) to the development of Phased Array Antenna Models to Improve Design Time and Quality The United...
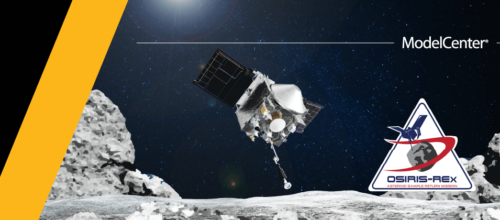
Lockheed Martin Space | OSIRIS-REx
Case Study
Lockheed Martin Space utilizes integrated Model-Based Systems Engineering (MBSE) to simulate the mission trajectory of the OSIRIS-REx spacecraft
Lockheed Martin Space utilizes integrated Model-Based Systems Engineering (MBSE) to simulate the mission trajectory of the OSIRIS-REx spacecraft On September 5, 2016, an Atlas V rocketed from Cape Canaveral carrying...
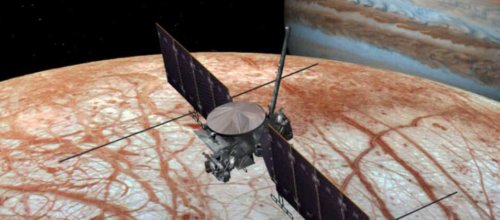
JPL
Case Study
Integration of Systems Models and Analytical Models Provides Order of Magnitude Time Savings
The National Aeronautics and Space Administration’s (NASA’s) Jet Propulsion Laboratory (JPL) is streamlining the space mission engineering process by integrating systems models that define the project architecture with the many...
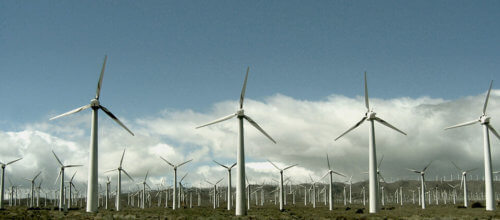
Wind Farm – Stanford
Case Study
Multilevel Optimization Reduces Cost of Building Wind Farm by Almost $4 Million
The Bobcat Bluff Wind Project, located in Archer City, Texas consists of 100 GE 1.5 MW turbines capable of producing up to 150 MW of electrical power. Like nearly all...
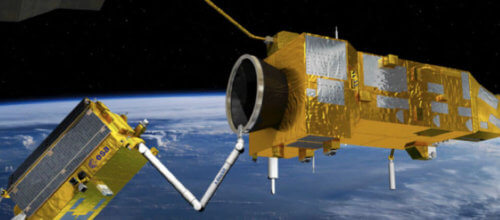
e.deorbit
Case Study
Integrating Systems Modeling with Simulation Helps Ensure Robust Space Debris Removal Mission
The European Space Agency (ESA) is studying an active debris removal mission called e.deorbit which is intended to capture an ESA-owned derelict satellite in low orbit and safely crash it...
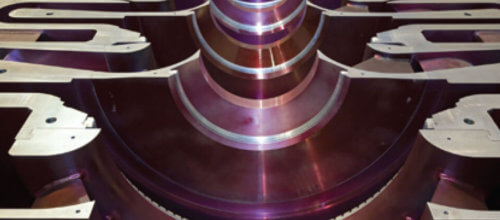
Centrifugal Compressor
Case Study
Analysis of Alternatives in ModelCenter Enables Rapid Selection of Optimal Centrifugal Compressor Configuration
Case Studies by Application
Webinar
Technical Papers
Phoenix Integration, Lockheed Martin
Authors: Hongman Kim, David Fried, Peter Menegay and Grant Soremekun, Christopher Oster
Authors: Nicolas Albarello et Hongman Kim